Generative AI is Boring
- Jake Browning
- Oct 26, 2023
- 4 min read
Updated: 4 days ago
Now that the dust has settled and the hype has died down (except on Twitter), we can give a verdict: generative AI is boring. We already know they can't reason, can't plan, only superficially understand the world, and lack any understanding of other people. And, as Sam Altman and Bill Gates have both attested, scaling up further won't fix what ails them. We're now able to evaluate what they are with fair confidence it won't improve much by doing more of the same. And the conclusion is: they're pretty boring.
This is easiest to see with generative images created by AI: lots of (hot) people standing 5-10 feet away, posing for the camera. Plenty of landscapes or buildings. And everything is absurdly baroque; the models excel at adding frills and needless details, filling every part of the image with excessive ornamentation. But, on the whole, it is mostly effective at well-lit static shots, taken straight on, with whatever is happening in the exact middle.

Occasionally, someone might produce a basic action shot, like someone jumping, running, or riding a dragon. But multiple, complex people interacting in an action shot? Really difficult. At best, the model just poses two objects in the same frame without letting them really touch, since they still struggle with object boundaries. If they do touch, the model's confusion becomes obvious. Here are two robot hands thumb wrestling:
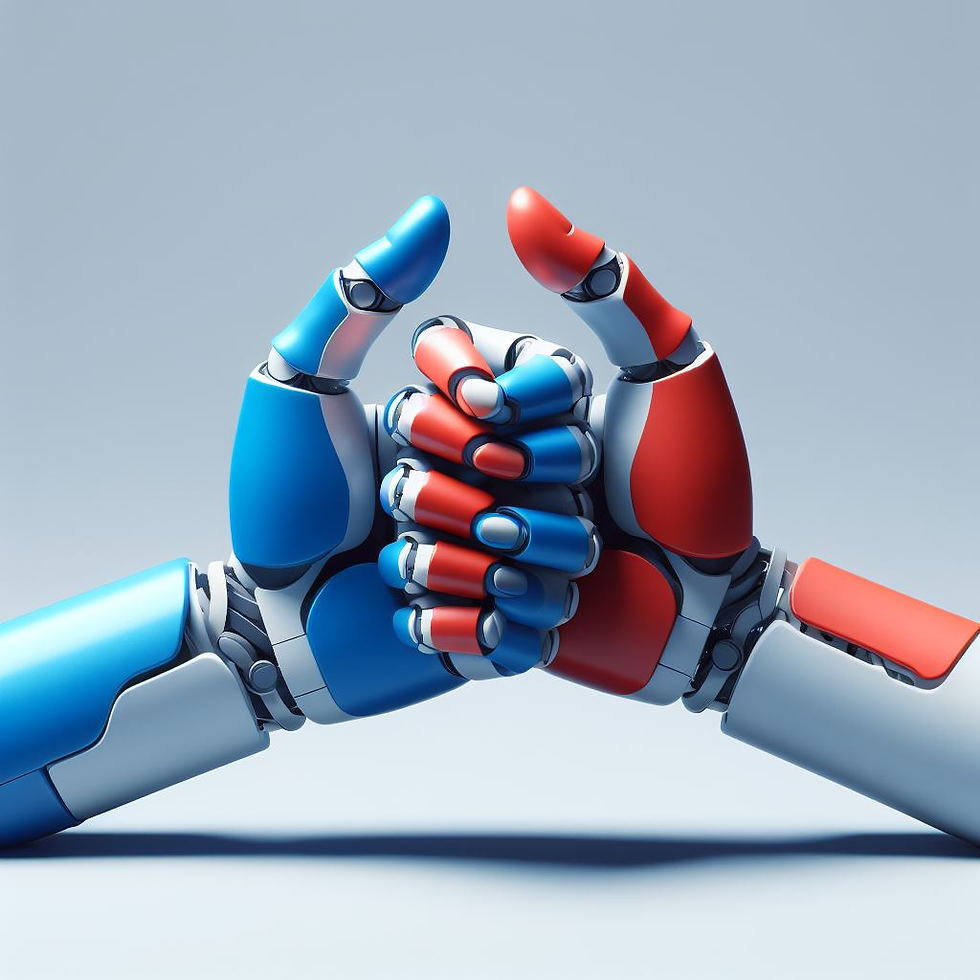
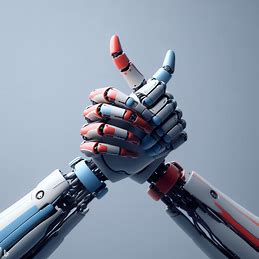

Thumb wrestling isn't even a difficult topic; there are plenty of photos of that. What about rare actions, like an ankle pick in wrestling? Or a complex one, like an old blue heeler chasing a silver fox towards a pink cactus? My results have the right objects in the frame (with the wrong colors, in some cases), but they don't get "chase" or "towards". How could they? You need to understand what objects are to understand object boundaries, and some sense of how they move to understand chasing and forward and back. The gap between what can be learned from images and how the world actually works still looms large.
Internet text is no different. The output of LLMs is too spotty and unreliable to be used for anything more than writing copy or generating common pieces of code. This isn't useless; people need search-optimized copy for webpages and simple code for their projects. But the hope of replacing journalists with bots hasn't panned out, and I'm fairly confident the usage in specific fields, like medicine, will fall off once the models are subjected to independent testing. In order to make these systems more reliable, they need to have a firmer grasp of what the texts are about--what all the words are referring to in the real world. Even when there does seem to be a shallow grasp of the underlying concept, the unreliability and unexpected mistakes suggest we shouldn't assume they know what we think they know.
But, worse, they are increasingly boring. As companies recognize the way harmful content by the bots reflects on their brand, they have increasingly hamstrung the models and encouraged them to provide increasingly generic answers. While this is great if the goal is to make them less harmful, there is no question they are becoming blander and discussing ever fewer topics. If it is too unreliable for factual questions, too stupid for problem-solving, and too generic to talk to, it isn't clear what their purpose is besides copy.
The increasingly boring nature of these models is, in its own way, useful. We now see more clearly the gap between what can be learned from the current type of generative models, even multimodal ones. They excel at the superficial stuff--like capturing a Wes Anderson aesthetic--but not improving on the more abstract stuff, on how things work and behave and interact. This is especially visible in the AI movie trailers, where fancy scenes and elaborate aliens briefly come into frame, but nothing ever happens--no space battles or sword fighting, just more blank faces staring at the camera and vista shots. The models are always stopping short of the interesting stuff--the stuff that is supposed to be in a movie trailer. Or, if they do interesting stuff, like in the Will Smith eating spaghetti bit, the glaring failure with boundaries and the fundamental misunderstanding of how eating works becomes too apparent.
Some hypeheads will argue we should just keep scaling up. But we can't. There isn't infinite internet text, infinite photos, infinite YouTube videos, and so on. Even if there was, the more pressing issue is that generative AI is obsessed with the superficial and still stuck with understanding the world according to what is easy to label and easy to recognize. We need models that are obsessed instead with the abstract--with ideas like object, movement, causation, and so on. Models aren't building up the world-models that are needed for getting to the more interesting use cases.*
This should be sobering. It isn't probably going to sober people just yet--not until Google admits Google AI Search isn't going to work and the startups promising the Moon get write-downs. But the writing is on the wall: the astronomical early successes in generative AI won't be repeated with more compute, so improvement is going to be minor using contemporary techniques. We need new ideas now, with more promise for understanding the underlying world, rather than just providing a lossy compression of the internet. The latter is just too boring to give people what they want.
*People sometimes suggest a language model can model the world, pointing to (limited) success at modelling the moves in a game like Othello. Games like this are "worlds" in only the loosest sense: they are two-dimensional, fully determinate spaces with minimal agents, no hidden information, strict rules, and operated in turns rather than in real-time. There is effectively no direct path from this kind of world-model to a genuine, dynamic world-model with different agents with their own intentions acting in unpredictable ways. This is a lesson Symbolic AI learned the hard way; see John Haugeland's criticism of "micro worlds," like the one used by SHRDLU, in AI: The Very Idea.
Generative AI is boring, but its use-cases will necessarily be discovered downstream anyway. By companies employing a technology, not hypesters promising it will do everything and anything.
With that kind of starting point (originally inspired by a certain CEO's boldfaced claim that "AI will lead to programmer unemployment in India") I wrote that we're headed to an AI slowdown if AI works as is advertised:
https://www.linkedin.com/pulse/were-headed-ai-slowdown-mikael-koivukangas-9rtwe
I would agree that current models have a poor understanding of reality; LLMs are like someone trapped in a white featureless box their entire life with great memory but no imagination, being read all the world's knowledge in a monotone and then being asked questions (and I am being generous here). But that doesn't mean they're not useful for a number of applications, from text summarization (my favorite usage right now) to semantic search. Yes, these are boring; yes, there is tons of hype; but they still represent a much more useful set of features than (say) cryptocurrency, and there is gold in them thar hills.
Amidst the crescendo of technological change that is reshaping our world, it's been boldly declared that generative AI, this groundbreaking frontier, has devolved into a realm of boredom, a monotonous echo chamber of superficiality. But this view, steeped in skepticism, perhaps overlooks the pulsating undercurrents of a revolution that is anything but mundane.
Consider the critique of generative AI as a purveyor of the ornamental, the excessively baroque. This is akin to condemning the early Impressionists for their apparent lack of finish, their supposedly chaotic brushstrokes. What if, in AI’s awkward attempts at capturing life, we are witnessing the infancy of a new intelligence, not its senility? The seemingly clumsy AI-generated images, the failure to grasp the complexity of motion…